Summary: Leonardo support for collaborative AI workflow in UX design | Udio: new AI generative music | Clear beats clever in UX | Hackathon to grow team skills: Example of an AI feature | AI enables new and more flexible business KPIs for UX work
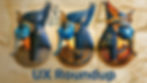
UX Roundup for April 12, 2024. (Midjourney)
Leonardo Launches Collaborative AI Workflow for UX Design
Leonardo, which is an AI tool for image generation, is launching an enterprise product called “Leonardo for Teams” with support for collaborative workflows in design.
If you work in a team, I encourage you to sign up for a trial (the service is currently in beta). The future of AI requires system thinking to support workflows and not just great usability for individual tasks. This again means that you should gain early experience with using AI across your team and not just for individual work.
To be honest, Leonardo is not my top image-generation AI tool. I use Midjourney, Ideogram, and Dall-E more often, but Leonardo is a solid 4th. In my recent study of the AI tools used the most by UX professionals, Leonardo came in as number 16. Solid placement, but not the very top.

A single designer working alone vs. a team working together. Since almost all UX design is done in a team, it’s important to gain experience with the best way of using AI in team projects, especially as AI adds enterprise features and team support. (Leonardo, using the “colorful scribbles” LoRA with weight 0.6)
New Generative Music Service: Udio
A new generative AI service for music launched this week: Udio.
Many people claim that the sound quality beats the previous leader in this space, Suno. On the other hand, in my initial experimentation, the lyrics generated by Udio are terrible. For example, I asked it for a song about my 10 heuristics, and the results are not worth publishing, whereas Suno produced decent songs based on nothing but that rough prompt.
In both cases, you’re better off producing the lyrics separately, maybe using ChatGPT or Claude 3 Opus for drafts, followed by manually editing the text before uploading it to the music-generation system.
Here’s a song I made about my 6th usability heuristic, “Recognition Rather than Recall,” using two different AI music services. To facilitate comparisons, I used the same lyrics for both versions and specified the same genre (country music).
Which version do you prefer? Tell me in the comments.
One pragmatic advantage of Suno is that it easily generates both soundtracks and video files. Udio does have a video option, but it is very slow. Initially, I only discovered the option to download audio files, which required me to use a terrible video editor (Adobe Premiere Elements) to make my video for upload to YouTube. Suno also generates longer songs of up to 2 minutes, whereas Udio limits initial creations to 32 seconds. (Both services allow you to extend the songs and offer rather good musical consistency in composing additional music and character persistency in the vocalization of the lyrics.)
The AI response time is poor for both music services, but Udio currently has atrocious response times: it often takes 10 minutes to generate 32 seconds of music. I hope this terrible performance is due to their servers being overloaded immediately after they launched to great publicity.
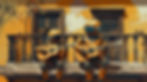
Two generative AI services serenade you with their songs: upstart Udio and long-established Suno. Which do you prefer? (Midjourney)
Clear Beats Clever
Adam Silver is a UX-slogan machine. Last month, I discussed his slogan “Boring UX Is Better UX.” Now, he coined a new slogan, “Clear UX Beats Clever UX” in his discussion of how to best show the label for an input field. Adam recommends just placing the label above the input field and eschewing clever design ideas such as making the label float or putting it inside the field to save space. (This latter design invariably causes problems as users start typing upon which the instructions vanish.)
The point that clear design is better than clever design generalizes far beyond this example. It’s almost always the case that users save much more time when interacting with a straightforward UI than when they use something that’s supposedly clever, but nonstandard.
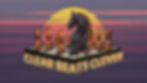
New UX slogan: “Clear Beats Clever.” (Ideogram)
Use Hackathons to Grow Team Skills — Example: Test a New AI Feature
An entertaining way to combine team building and skill building is to run small hackathons to try out new features. For a small feature, you can run the complete hackathon in an hour, for example over lunch.
This idea is particularly suited for growing your team’s awareness of the growing capabilities of AI tools. There’ll be something new to try every week!
Here’s an example of experimenting with Midjourney’s feature for character consistency in image creation. For any image prompt, you can add the parameter --cref <URL> to use an existing image as a character reference. In typical Midjourney style, “character reference” is abbreviated cref and used in a rather obscure manner. However, if you use the web UI — still in limited alpha release — you get to see a tiny thumbnail of your character.
It is essential to set a defined task for your team to achieve. The hackathon is not about playing around with the tool. It’s about testing it out with a goal in mind. No different than setting a task for the participants in a usability study.
In this case, the task was to create the panels for a short comic strip for my article Does AI Numb the Brain? The analogy visualized in the comic strip is that if a formerly-strong warehouse worker starts driving a forklift all day, it’ll be necessary to join a gym to retain muscle tone.
Here are the 3 panels I created in a hackathon. Midjourney, in fact, provided excellent character consistency.
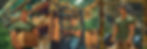
3 panels from a comic strip about a warehouse worker becoming weak from no longer lifting heavy boxes at work. (Midjourney)
I ended up recreating the comic strip in another tool for the sake of stylistic consistency with a second comic strip in the same article about the adventures of a knowledge worker who starts using AI to do complex data analysis.
If you want to try your hand at this task in your own hackathon, here’s the scenario:
Character description: Boris is a muscular warehouse worker with short brown hair and wearing a tight green t-shirt.
Panel 1: Boris is working in a warehouse carrying big, heavy boxes around himself.
Panel 2: The warehouse gets forklift trucks, and Boris has a great time driving the forklift without needing to carry the heavy boxes himself.
Panel 3: Now that he is no longer carrying heavy boxes, Boris gets weak. He joins a gym and starts lifting weights. This makes him strong again!
The point is not the goal of creating a comic strip or the exact scenario for the strip. The point is to define a task in advance and set all your team members to work, trying to perform the task. Hilarity will ensue, especially for any media creation task, as hackathon participants show off their preliminary progress and learn from each other’s successes and mistakes.
New AI-Derived KPIs Enable More Flexible Assessment of the Business Impact of UX Work
MIT Sloan School and the Boston Consulting Group have published an interesting report on how one can use AI to define new types of KPI measures (key performance indicators) that would have been impossible or too difficult to collect with traditional methods. They define 5 areas in which AI can advance KPI in business:
Descriptive KPIs: AI synthesizes historical and current data to provide deeper insights into performance gaps and their root causes. This enables identifying critical interdependencies between different KPIs. The benefit is getting a more holistic understanding of the customer experience and how it impacts overall business metrics.
Predictive KPIs: By leveraging AI's ability to identify complex patterns, predictive KPIs can reliably forecast future performance in ways humans cannot. This visibility into potential outcomes allows teams to proactively design experiences optimized for key leading indicators.
Prescriptive KPIs: AI-powered prescriptive KPIs recommend specific actions to address performance gaps. For UX, this could translate into AI suggesting UX/UI improvements or personalization strategies to boost metrics like engagement, conversion, or customer lifetime value.
Linking KPIs: AI helps establish relationships between seemingly unrelated KPIs across business functions. UX professionals could leverage this to demonstrate how UX metrics connect to and influence critical business KPIs.
Experimentation: Techniques like digital twins allow safely simulating and testing the impact of changes on KPIs before deploying them. This approach may be more useful for non-UX KPIs, since it’s not possible to fully simulate user behaviors without engaging real humans.
Overall, AI enables transitioning KPIs from static benchmarks to dynamic, predictive tools. For UX, this means getting richer insights, connecting UX to business outcomes (something that was sorely lacking in the past), and continuously optimizing the user experience based on forward-looking metrics. According to the report, only 34% of companies currently use AI to define new KPIs, but this percentage is expected to increase.
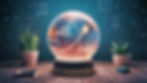
AI can create new predictive KPI metrics by flexibly combining large amounts of disparate data, giving us a new crystal ball to improve the business profitability of UX design. (Ideogram)
Let’s make up a few possible examples of applying these ideas to new UX metrics.
Descriptive KPIs:
Time Spent on Key User Flows: Tracks the average time users spend on critical user flows. AI processes user interaction data to calculate time spent and identify bottlenecks.
Interaction Rate with Key UI Elements: Measures the percentage of users who interact with important UI elements. AI analyzes click and touch data to determine interaction rates.
User Confusion Index: Assesses the level of user confusion based on factors like hesitation, backtracking, and error clicks. AI combines multiple behavioral signals to calculate a confusion score.
UI Consistency Score: Evaluates the consistency of UI elements across the product. AI compares UI elements to design system standards to calculate a consistency score.
Predictive KPIs:
Projected User Retention Rate: Predicts the percentage of users likely to continue using the product over a given period. AI analyzes behavioral indicators of retention to make projections.
Anticipated User Support Request Volume: Forecasts the volume of user support requests based on factors like user confusion and error rates. AI models estimate support demand based on UX-related signals.
Prescriptive KPIs:
Recommended UI Simplification Index: Suggests areas of the UI that could be simplified to improve usability. AI identifies complexity hotspots and recommends reduction targets.
Suggested Onboarding Flow Optimization Score: Prescribes improvements to the user onboarding flow based on completion rates and drop-off points. AI pinpoints opportunities to streamline onboarding.
Linked KPIs:
User Engagement Impact on Revenue: Quantifies the relationship between user engagement metrics and revenue. AI models estimate the revenue impact of changing engagement levels.
Usability Influence on Customer Retention: Measures the effect of usability improvements on customer retention rates. AI analyzes the correlation between usability scores and retention.
User Satisfaction Correlation with Brand Loyalty: Assesses the link between user satisfaction and brand loyalty. AI examines the relationship between satisfaction ratings and loyalty indicators.
UI Consistency Impact on Support Costs: Quantifies the cost savings from improving UI consistency. AI estimates the support cost reduction attributable to consistency enhancements.
Onboarding Effectiveness Relation to Feature Adoption: Measures the correlation between onboarding completion rates and feature adoption. AI analyzes the relationship between these two metrics.
These were just my initial ideas, and not all of them may be easy to implement in a valid manner with current AI. Still, my list may help you get more idea for ways that AI can define trackable usability and UX KPIs for your own business that reach far beyond the primitive metrics we’re currently using.